Game Theory of Reasonable Artificial Intelligence (Part 2)
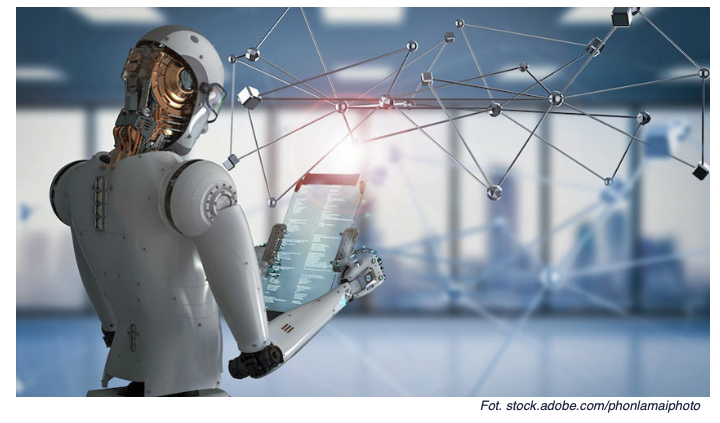
Introduction
The ability to work out how artificial intelligence (AI) will be "thinking" is essential for effective AI and human interactions, be they cooperative (reasonable) or competitive (rational). Constructing AI in a way to make it work out optimal strategies that balance cooperation and competition remains a central puzzle in AI research.
Game Theory of Reasonable Artificial Intelligence
This note argues for introducing a model of ‘theory of cooperative (reasonable) AI’, namely, how AI represent the intentions and goals of others to optimise our (AI and humans) mutually beneficial interactions. This note draws on ideas from game theory to provide a ‘game theory of cooperative (reasonable) AI’’. First, we consider the representations of goals in terms of value functions that are prescribed by utility or rewards. Critically, the joint value functions and ensuing behaviour are optimised recursively, under the assumption that I represent your value function, your representation of mine, your representation of my representation of yours, and so on ad infinitum. However, if we assume that the degree of recursion is bounded, then players need to estimate the opponent's degree of recursion (i.e., sophistication) to respond optimally. This induces a problem of inferring the opponent's sophistication, given behavioural exchanges. We show it is possible to deduce whether players make inferences about each other and quantify their sophistication on the basis of choices in sequential games. This rests on comparing generative models of choices with, and without, inference. Model comparison is demonstrated using simulated and real data from a ‘stag-hunt’. Finally, we note that exactly the same sophisticated behaviour can be achieved by optimising the utility function itself (through prosocial utility), producing unsophisticated but apparently altruistic agents. This may be relevant ethologically in hierarchal game theory and coevolution.
Conclusion
Exploiting insights from computer science and human behavioural economics, we suggest a model of ‘theory of reasonable AI’ using ‘recursive sophistication’ in which AI's model of human goals includes a model of human model of AI's goals, and so on ad infinitum. By studying experimental data in which people played a computer-based group hunting game, we show that the model offers a good account of individual decisions in this context, suggesting that such a formal ‘theory of mind’ model can cast light on how people build internal representations of other people in social interactions.
Comments